Harnessing cutting-edge artificial intelligence techniques to offer unparalleled accuracy in predictive outcomes.
Our proprietary algorithms enable us to create nonlinear, dynamic models of credit decisioning that significantly outperform traditional underwriting methods.
The inception of underwrite.ai traces back to the medical field, where founder Marc Stein worked on correlating DNA microarray data with the probability of prostate cancer. This project required the development of machine learning techniques capable of handling extensive and complex data sets—25,000 genes across just 25 sample cases.
The success of this project sparked the idea to apply these sophisticated algorithms to other domains, particularly consumer and small business lending. Just as nonlinear approaches have transformed medical diagnostics, they have the potential to revolutionize credit modeling by providing more accurate, dynamic assessments of risk.
Marc Stein, the founder of underwrite.ai, brings over 20 years of experience in underwriting systems for major financial institutions, including JP Morgan Chase (consumer lending), Freedom Debt Relief (debt settlement), and LeaseQ (equipment leasing).
His deep expertise in developing robust underwriting systems forms the backbone of underwrite.ai's advanced credit risk models.
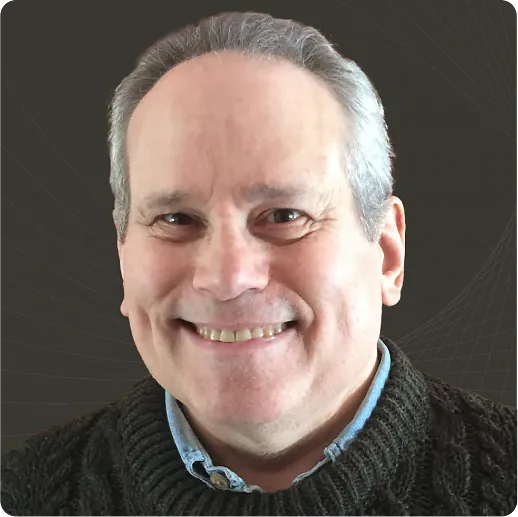
At underwrite.ai, our mission is to expand credit availability to new markets and underserved populations around the world
We believe that if we hold ourselves to the highest ethical standards, regulatory compliance becomes simple. We also believe that business ethics applies to fundamental business models as well. We do not charge clients for data studies or to generate initial models.
Before we take any money from a client, we ensure that the client knows how much lift our model will provide and that using underwrite.ai will increase profits beyond our costs.
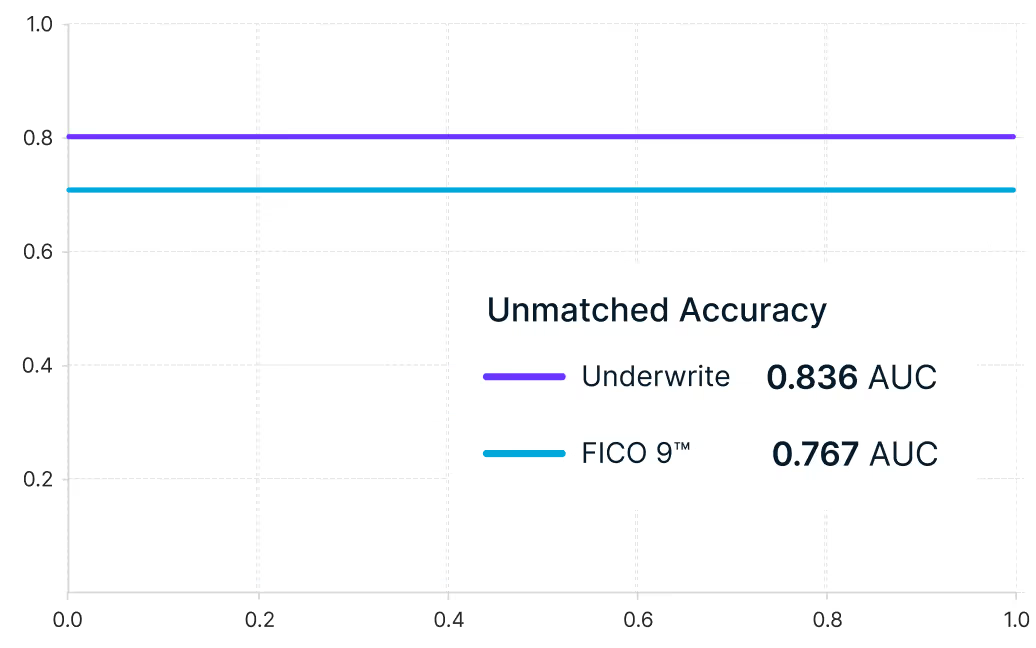
Explore our resources about the use of artificial intelligence in financial services
Start for Free Today
Get in touch to set up your custom model and experience underwrite.ai free of charge for 30 days.